Intro
History
Current Approach
Conversations and emotions
Single modal vs multi-modality
Text-based emotions from natural language
Vocal tone based emotions
Our experience and focus
Measuring emotional connection
Data-sets and accuracy
Language of emotions
Emotional patterns
Vision for next decade
Emotion characteristics
Can emotions be tracked across different languages and cultures?
Is emotion recognition in conversation (ERC) reliable?
What is difference between basic emotions and social emotions?
Social Intelligence
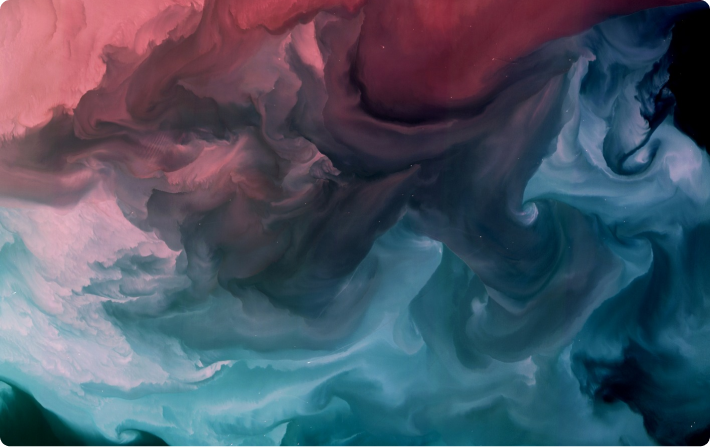
Social intelligence is the cornerstone of relationships. It includes far wider range of skills than emotional intelligence. It involves being good at communicating, listening, public speaking, understanding social norms, and recognizing what motivates others and how they feel. It's about understanding the unspoken rules of the game and reading the emotional cues others send out.
The starting point - emotions. Emotions are a natural and original part of us. Without them we are broken. We might be calm one moment, anxious the next and uplifted and happy the next.
These states serve a purpose in driving our behavior, navigating social interactions, and even aiding in faster or more driven decision-making. Therefore, understanding the nature of these states and how to measure them can help us improve in these areas:
In psychology, two approaches are used to describe emotional states: Categorical and Dimensional. The latter is the more modern method since it captures more depth and variety of different emotional experiences and the energy (arousal) they carry.
These states serve a purpose in driving our behavior, navigating social interactions, and even aiding in faster or more driven decision-making. Therefore, understanding the nature of these states and how to measure them can help us improve in these areas:
- Our own skills in managing emotions
- Social interaction skills
- Their influence on decision-making
In psychology, two approaches are used to describe emotional states: Categorical and Dimensional. The latter is the more modern method since it captures more depth and variety of different emotional experiences and the energy (arousal) they carry.
History
Psychologist Edward Thorndike pioneered the concept of social intelligence in his 1920 article, “Intelligence and Its Uses,” published in Harper's Magazine. He differentiated social intelligence from abstract and mechanical intelligence, defining it as the ability to comprehend and navigate interpersonal dynamics effectively.
Affective computing and sentiment analysis have been continually developing since 1997. The key component is emotion recognition, which aims to identify meaningful patterns within a conversation. Some foundational methods for tracking this information in the context of a conversation include analyzing facial expressions, tone of voice, speech segment semantics, and lexicon.
The main areas of interest for using this technology are in Al customer service, sales, automotive technologies, education, and health and wellness. However, mastering this task remains challenging due to the complexity of variables involved, such as individual human differences, gender, social baselines, social coherence, and data quality, among others.
In 1971, Ekman and Friesen described six basic and universal emotions: happiness, sadness, anger, surprise, disgust, and fear. More recently, academics have reduced these to four: fear, happiness, sadness, and anger. An additional “Neutral” emotion has also been recognized. The current approach is the Circumplex model, developed by James A. Russell in 1980.
Its basic approach uses two main metrics: valence (pleasurable or unpleasurable) and arousel (low to high energy) in a two-dimensional model. This updated approach helps to further understand how emotions can be measured. Since people often argue that emotions are subjective experiences, this model represents the basic idea that positive emotions, ike joy and happiness, are considered pleasant, while those on the negative end, like sadness and anger, are considered unpleasant. However, it does not regard negative emotions as bad or unfavorable.
Currently, this model is considered universally applicable across different cultures. Emotional states can be described in familiar terms such as happy, angry, and sad. However, in the context of social conversation, there can be emphasis on altered version of sadness. For example, projecting emotions to adapt to the client can be expressed as empathy. Similarly, anger can be expressed as dominance and passion to persuade the client about a product and challenge the validity of objections in a sales process.
Affective computing and sentiment analysis have been continually developing since 1997. The key component is emotion recognition, which aims to identify meaningful patterns within a conversation. Some foundational methods for tracking this information in the context of a conversation include analyzing facial expressions, tone of voice, speech segment semantics, and lexicon.
The main areas of interest for using this technology are in Al customer service, sales, automotive technologies, education, and health and wellness. However, mastering this task remains challenging due to the complexity of variables involved, such as individual human differences, gender, social baselines, social coherence, and data quality, among others.
In 1971, Ekman and Friesen described six basic and universal emotions: happiness, sadness, anger, surprise, disgust, and fear. More recently, academics have reduced these to four: fear, happiness, sadness, and anger. An additional “Neutral” emotion has also been recognized. The current approach is the Circumplex model, developed by James A. Russell in 1980.
Its basic approach uses two main metrics: valence (pleasurable or unpleasurable) and arousel (low to high energy) in a two-dimensional model. This updated approach helps to further understand how emotions can be measured. Since people often argue that emotions are subjective experiences, this model represents the basic idea that positive emotions, ike joy and happiness, are considered pleasant, while those on the negative end, like sadness and anger, are considered unpleasant. However, it does not regard negative emotions as bad or unfavorable.
Currently, this model is considered universally applicable across different cultures. Emotional states can be described in familiar terms such as happy, angry, and sad. However, in the context of social conversation, there can be emphasis on altered version of sadness. For example, projecting emotions to adapt to the client can be expressed as empathy. Similarly, anger can be expressed as dominance and passion to persuade the client about a product and challenge the validity of objections in a sales process.
Current Approach
To fully explore modern trends in valances, let's delve deeper into their characteristics. In emotion detection, valence refers to whether an event, object, or situation is inherently attractive (positive valence) or repulsive (negative valence). Within emotion detection, valence helps categorize emotions by their pleasantness or unpleasantness in response to the emotional trigger.
In emotion detection technologies and studies, valence is typically combined with arousal (the level of calmness or excitement) to create a two-dimensional model of emotions. This model helps us understand the complexity of human emotions by distinguishing between different states, such as happiness (high valence and high arousal), sadness (low valence and low arousal), anger (low valence and high arousal), and relaxation (high valence and low arousal).
To sum up, Valence in other words how pleasurable an emotion, and activation with how much energy, referred as arousal.
Valence, in other words, describes how pleasurable an emotion is, while arousal refers to the level of energy associated with it. Emotion expressions are a crucial form of communication in interpersonal relationships. Our current day research investigates how these expressions influence relationship building, persuasion, and decision-making during conversations.
Until today, much research has explored the concept of "productive emotions," examining how these mental experiences influence well-being. Our research delves into social psychology, specifically how emotions impact social phenomena: their effects on our thoughts, behaviors, trust, decision-making, persuasion, and social skills.
In emotion detection technologies and studies, valence is typically combined with arousal (the level of calmness or excitement) to create a two-dimensional model of emotions. This model helps us understand the complexity of human emotions by distinguishing between different states, such as happiness (high valence and high arousal), sadness (low valence and low arousal), anger (low valence and high arousal), and relaxation (high valence and low arousal).
To sum up, Valence in other words how pleasurable an emotion, and activation with how much energy, referred as arousal.
Valence, in other words, describes how pleasurable an emotion is, while arousal refers to the level of energy associated with it. Emotion expressions are a crucial form of communication in interpersonal relationships. Our current day research investigates how these expressions influence relationship building, persuasion, and decision-making during conversations.
Until today, much research has explored the concept of "productive emotions," examining how these mental experiences influence well-being. Our research delves into social psychology, specifically how emotions impact social phenomena: their effects on our thoughts, behaviors, trust, decision-making, persuasion, and social skills.
Conversations and emotions
The topic of emotion detection is often criticized for being subjective, as everyone interprets emotions differently. You might wonder, "Who can objectively label emotions?" Some post-modern thinkers even claim that everything is subjective and there is no objective truth in the realm of emotions. However, this view is not entirely accurate. Despite the hidden motives behind this ideology, we can counter it with our own free will. By observing the world through our own eyes and making personal value judgments, we can contribute to a consensus within a small community to establish objective truth. For example, this consensus is how nations develop a common language and culture.
When it comes to emotions, for example, feeling happy while conversing with a friend can be considered a social emotion which we define as “social emotions”. In contrast, experiencing happiness while alone and engrossed in creative work is not, as it lacks direct interaction with another person. However, upon closer examination, it becomes apparent that most emotions are linked to our relationships with others and are frequently expressed through communication.
Furthermore, a subset of emotions can be categorized as "productive emotions" as they are not intrinsically linked to social contexts. Fear of heights is one example. This distinction differentiates our approach from that of some contemporary scholars. We focus on investigating emotions and their impact on interpersonal relationships within social contexts. Conversation is the direct medium through which these social emotions are conveyed.
In reality, the emotions expressed during conversations are far more nuanced than those often depicted in television shows and social media. The subtle nature of emotions expressed in everyday conversations significantly complicates the task of accurate emotion detection. Furthermore, the intensity of social emotions exhibits dynamic fluctuations across different conversational contexts. Typically, conversations are characterized by periods of emotional neutrality interspersed with brief, yet potent emotional peaks.
When it comes to emotions, for example, feeling happy while conversing with a friend can be considered a social emotion which we define as “social emotions”. In contrast, experiencing happiness while alone and engrossed in creative work is not, as it lacks direct interaction with another person. However, upon closer examination, it becomes apparent that most emotions are linked to our relationships with others and are frequently expressed through communication.
Furthermore, a subset of emotions can be categorized as "productive emotions" as they are not intrinsically linked to social contexts. Fear of heights is one example. This distinction differentiates our approach from that of some contemporary scholars. We focus on investigating emotions and their impact on interpersonal relationships within social contexts. Conversation is the direct medium through which these social emotions are conveyed.
In reality, the emotions expressed during conversations are far more nuanced than those often depicted in television shows and social media. The subtle nature of emotions expressed in everyday conversations significantly complicates the task of accurate emotion detection. Furthermore, the intensity of social emotions exhibits dynamic fluctuations across different conversational contexts. Typically, conversations are characterized by periods of emotional neutrality interspersed with brief, yet potent emotional peaks.
Single modal vs multi-modality
Early AI emotion detection systems were primarily designed to process single-modal inputs, such as images, videos, text, or audio. Numerous companies specialized in refining these individual capabilities, often becoming acquisition targets for larger corporations seeking to integrate these specialized domains into their broader offerings. For example, the acquisition of Affectiva by SmartEyes in 2021 aimed to enhance emotion detection capabilities specifically for the automotive industry. This focused approach to emotion detection, however, has limitations when applied to the complexities of human interaction.
The current state-of-the-art in emotion recognition involves a multimodal approach, which seeks to enhance accuracy by combining information from multiple channels. For instance, while anger is often more reliably detected through vocal tone, happiness is typically better discerned from facial expressions. This multimodal approach is essential as certain emotions are more effectively conveyed through specific modalities. For example, it is challenging to convincingly express anger through the phrase "Thank you, have a nice day," highlighting the limitations of relying solely on textual content. Consequently, sentiment analysis, which evaluates the emotional tone of text, plays a crucial role in supplementing multimodal emotion detection systems and improving their overall accuracy.
Future research in emotion detection is poised to explore a vast array of modalities, extending beyond traditional methods. This includes physiological signals such as millimeter-wave-based heart rate monitoring (heart waves), brain activity patterns (EEG), heart rate variability (pulse), electrocardiograms (ECG), and skin conductance. Additionally, biochemical markers (hormones) and linguistic analyses, encompassing lexical, semantic, and even narrative-level (symbolic semantics) components, will be investigated. This comprehensive approach aims to provide a more holistic understanding of human emotions and their underlying mechanisms.
The current state-of-the-art in emotion recognition involves a multimodal approach, which seeks to enhance accuracy by combining information from multiple channels. For instance, while anger is often more reliably detected through vocal tone, happiness is typically better discerned from facial expressions. This multimodal approach is essential as certain emotions are more effectively conveyed through specific modalities. For example, it is challenging to convincingly express anger through the phrase "Thank you, have a nice day," highlighting the limitations of relying solely on textual content. Consequently, sentiment analysis, which evaluates the emotional tone of text, plays a crucial role in supplementing multimodal emotion detection systems and improving their overall accuracy.
Future research in emotion detection is poised to explore a vast array of modalities, extending beyond traditional methods. This includes physiological signals such as millimeter-wave-based heart rate monitoring (heart waves), brain activity patterns (EEG), heart rate variability (pulse), electrocardiograms (ECG), and skin conductance. Additionally, biochemical markers (hormones) and linguistic analyses, encompassing lexical, semantic, and even narrative-level (symbolic semantics) components, will be investigated. This comprehensive approach aims to provide a more holistic understanding of human emotions and their underlying mechanisms.
Text-based emotions from natural language
Traditional emotion detection in natural language processing often relies on simplistic word or phrase classification. However, the dynamic nature of conversational exchanges presents a more complex challenge. A single utterance can encapsulate multiple, often contradictory, emotional states. For instance, a shared story might evoke both sadness and joy, while the subsequent response indicates that sadness is the predominant emotion. Moreover, identifying the cause of the sadness, rather than simply recognizing its presence, is crucial for a deeper understanding of the emotional landscape. This necessitates sophisticated models that can account for the intricate interplay of emotions within conversational context.
Vocal tone based emotions
Our scientific approach is firmly rooted in the foundational theories proposed by pioneering researchers such as Paul Ekman (1934). His work laid the groundwork for our understanding of fundamental human emotions. However, we also maintain a receptive stance towards unproven methodologies, including the provocative theories advanced by contemporary scientists like Lisa Feldman Barrett (1963). Her work questions established wisdom and offers different perspectives on the nature of emotions. By integrating these diverse theoretical frameworks, we aim to create a comprehensive and nuanced understanding of the emotional experience.
Our research has identified at least three universal core emotions across the whole world, supplemented by a more intricate spectrum of social emotions. While tonal and cultural nuances can complicate the interpretation of these social emotions, text-based lexical analysis provides a valuable tool for verifying their impact and enhancing the overall reliability of our findings. Importantly, this combined approach underscores the objective nature of emotions, challenging the notion that they are subjective experiences.Our research indicates a universal foundation of human emotions shared across cultures. These core emotional experiences, such as happiness, sadness, anger represent the universal aspect of human emotion. However, cultural variations significantly influence the baseline levels or intensities at which these emotions are expressed and experienced. For instance, a statistically typical Finnish individual might exhibit a lower baseline for expressing happiness compared to their Italian counterpart. This suggests that while the emotional experiences themselves are universal, cultural factors shape the frequency, intensity, and outward expression of these emotions.In essence, universal emotions represent the core emotional repertoire shared by all humans, while baseline levels reflect the culturally influenced average intensity or frequency of these emotions within a particular population.
Our research has identified at least three universal core emotions across the whole world, supplemented by a more intricate spectrum of social emotions. While tonal and cultural nuances can complicate the interpretation of these social emotions, text-based lexical analysis provides a valuable tool for verifying their impact and enhancing the overall reliability of our findings. Importantly, this combined approach underscores the objective nature of emotions, challenging the notion that they are subjective experiences.Our research indicates a universal foundation of human emotions shared across cultures. These core emotional experiences, such as happiness, sadness, anger represent the universal aspect of human emotion. However, cultural variations significantly influence the baseline levels or intensities at which these emotions are expressed and experienced. For instance, a statistically typical Finnish individual might exhibit a lower baseline for expressing happiness compared to their Italian counterpart. This suggests that while the emotional experiences themselves are universal, cultural factors shape the frequency, intensity, and outward expression of these emotions.In essence, universal emotions represent the core emotional repertoire shared by all humans, while baseline levels reflect the culturally influenced average intensity or frequency of these emotions within a particular population.
Our experience and focus
Unlike traditional academic research, our primary objective is to deliver immediate, actionable value to end-users such as managers, agents, and leaders. This necessitates a highly iterative scientific approach that is adaptable to exploring novel problem-solving strategies. For example, during the development of our emotion models, we encountered a significant challenge: contempt was initially misclassified as happiness, rendering the output unreliable. However, through rapid experimentation, we discovered that contempt is a highly social emotion that is difficult to accurately detect through vocal cues. This led to the strategic decision to exclude contempt from our initial focus and concentrate on emotions with more reliable indicators.
The approach prioritizes practical application over theoretical exploration, enabling us to rapidly refine our models and deliver tangible benefits to our users.
Our methodology is continually evolving, drawing upon our expertise in data science, mathematics, and design. We love good conversations and critical thinking. We aim to empower individuals with the ability to learn and grow rapidly through the use of Pitch Patterns. Our approach is grounded in a three-stage learning journey.
Initially, we focus on building a strong foundation by familiarizing users with key concepts, methodologies, and performance metrics. Second, we emphasize the disciplined practice to drive metric improvement. This phase, akin to the challenges faced by young professionals, demands dedication and perseverance. Finally, mastery is achieved through sustained performance excellence and a deep-rooted passion for sharing knowledge with others. These mastery-level users become invaluable assets, capable of rapidly imparting their expertise to new learners.
By combining rigorous data analysis with a human-centered design approach, we create a dynamic learning experience that yields tangible results. Our goal is to equip individuals with the skills and confidence to excel in their communication endeavors.
The approach prioritizes practical application over theoretical exploration, enabling us to rapidly refine our models and deliver tangible benefits to our users.
Our methodology is continually evolving, drawing upon our expertise in data science, mathematics, and design. We love good conversations and critical thinking. We aim to empower individuals with the ability to learn and grow rapidly through the use of Pitch Patterns. Our approach is grounded in a three-stage learning journey.
Initially, we focus on building a strong foundation by familiarizing users with key concepts, methodologies, and performance metrics. Second, we emphasize the disciplined practice to drive metric improvement. This phase, akin to the challenges faced by young professionals, demands dedication and perseverance. Finally, mastery is achieved through sustained performance excellence and a deep-rooted passion for sharing knowledge with others. These mastery-level users become invaluable assets, capable of rapidly imparting their expertise to new learners.
By combining rigorous data analysis with a human-centered design approach, we create a dynamic learning experience that yields tangible results. Our goal is to equip individuals with the skills and confidence to excel in their communication endeavors.
Measuring emotional connection
The dynamic nature of conversation profoundly influences the experience and expression of emotions. Unlike passive consumption of media, such as watching a film, where emotions are primarily elicited within an individual, dialogue involves a complex interplay of emotional projection and response. Participants actively co-create emotional states, resulting in frequent overlaps and nuances. When emotional resonance between individuals is high, we might describe this as empathy; conversely, a low degree of overlap can indicate apathy.
Our system excels at tracking these intricate emotional dynamics by identifying key markers of emotional mirroring. These markers include instances of shared empathy, positive emotional reciprocity, or a failure to resonate with projected emotions. By analyzing these patterns, we can gain valuable insights into the conversational dynamics and the emotional connections between participants.
Our system excels at tracking these intricate emotional dynamics by identifying key markers of emotional mirroring. These markers include instances of shared empathy, positive emotional reciprocity, or a failure to resonate with projected emotions. By analyzing these patterns, we can gain valuable insights into the conversational dynamics and the emotional connections between participants.
Data-sets and accuracy
Similar to human infants who acquire emotional understanding through familial and societal interactions, our AI undergoes a rigorous training process. To mitigate biases and ensure accuracy, we employ a robust validation framework. Our AI model is trained on an extensive dataset incorporating diverse modalities such as video, audio, and textual data. To establish a reliable benchmark, we utilize a globally recognized dataset featuring professional actors' meticulously recorded emotional expressions. A diverse panel of human experts cross-validates these emotional projections to guarantee accuracy.
Voice tone is a particularly potent indicator of emotion, often conveying up to 38% of emotional information, with certain emotions, such as anger, primarily expressed vocally. Our tone-of-voice model, tested on academic datasets, achieves an impressive accuracy rate of 90.25%.
Voice tone is a particularly potent indicator of emotion, often conveying up to 38% of emotional information, with certain emotions, such as anger, primarily expressed vocally. Our tone-of-voice model, tested on academic datasets, achieves an impressive accuracy rate of 90.25%.
The practice of labeling emotions is a common approach adopted by psychologists and parents alike in teaching emotional literacy. However, the precise vocabulary of emotions remains a subject of intense debate due to the subjective nature of emotional experiences. For instance, what one individual perceives as a complex and rare emotion of joy, another might experience daily and categorize simply as happiness, inspiration, or hope. This highlights the challenge of accurately capturing the nuanced and individual nature of emotions through language.
The complexity arises from the fact that emotions are often multifaceted and intertwined, making it difficult to isolate and define them with precision. Furthermore, cultural and linguistic differences can significantly impact the way emotions are expressed and understood, further complicating the issue.
Our research indicates a strong correlation between the emotion of anger and the concept of dominance. For instance, the phrases "I was angry with my son" and "I was dominating over my son" may appear distinct but share underlying similarities. While the former implies an uncontrolled emotional outburst, the latter suggests a more calculated assertion of power. Despite these nuances, both expressions convey negative or unpleasant affect and are frequently associated with anger within our model.
Crucially, the context of a conversation significantly influences the likelihood of interpreting an utterance as anger or dominance. In hierarchical relationships, dominance is more likely to be perceived, as individuals in positions of authority often exert control without necessarily expressing overt anger. Conversely, anger is more commonly attributed to interactions between equals. Our findings underscore the importance of considering conversational context when analyzing emotional states.
The complexity arises from the fact that emotions are often multifaceted and intertwined, making it difficult to isolate and define them with precision. Furthermore, cultural and linguistic differences can significantly impact the way emotions are expressed and understood, further complicating the issue.
Our research indicates a strong correlation between the emotion of anger and the concept of dominance. For instance, the phrases "I was angry with my son" and "I was dominating over my son" may appear distinct but share underlying similarities. While the former implies an uncontrolled emotional outburst, the latter suggests a more calculated assertion of power. Despite these nuances, both expressions convey negative or unpleasant affect and are frequently associated with anger within our model.
Crucially, the context of a conversation significantly influences the likelihood of interpreting an utterance as anger or dominance. In hierarchical relationships, dominance is more likely to be perceived, as individuals in positions of authority often exert control without necessarily expressing overt anger. Conversely, anger is more commonly attributed to interactions between equals. Our findings underscore the importance of considering conversational context when analyzing emotional states.
Emotional patterns
The basic building blocks of emotions are called affects. These are small negative or positively directed states that we feel, sort of like pleasure or pain. These subtle, often subconscious reactions serve as the raw material from which our emotional experiences are created. Consider the stark contrast between a stranger's accidental bump followed by a genuine apology and a hostile glare accompanied by a menacing remark. The former elicits curiosity and potential interest, while the latter triggers discomfort and a defensive posture.
Social emotions, on the other hand, are more enduring states that emerge as affects become intertwined with cognitive processes. They are colored by our interpretations, memories, and community. A serene natural setting, for example, may evoke feelings of peace and tranquility. Yet, the precise factors contributing to this emotional response are often complex and difficult to articulate, as they are influenced by a multitude of personal and contextual variables.
While emotions are inherently personal, certain emotional experiences exhibit universal characteristics. Shame, for example, is a complex emotion that manifests differently for individuals, yet there are shared triggers across cultures, such as financial embarrassment or public mishap.
However, unlike overtly expressed emotions like anger or joy, shame often resides in the realm of private experience, making it challenging to detect through vocal cues. Pitch Patterns offers a unique approach by identifying subtle emotional patterns within speech, providing valuable insights for individuals seeking to improve their emotional intelligence.
By analyzing these patterns, individuals can gauge the effectiveness of their emotional responses and identify areas for growth. Whether it's enhancing emotional regulation, refining empathetic responses, or mastering the art of emotional expression, Pitch Patterns empowers users to become more adept at navigating the complexities of human interaction.
Social emotions, on the other hand, are more enduring states that emerge as affects become intertwined with cognitive processes. They are colored by our interpretations, memories, and community. A serene natural setting, for example, may evoke feelings of peace and tranquility. Yet, the precise factors contributing to this emotional response are often complex and difficult to articulate, as they are influenced by a multitude of personal and contextual variables.
While emotions are inherently personal, certain emotional experiences exhibit universal characteristics. Shame, for example, is a complex emotion that manifests differently for individuals, yet there are shared triggers across cultures, such as financial embarrassment or public mishap.
However, unlike overtly expressed emotions like anger or joy, shame often resides in the realm of private experience, making it challenging to detect through vocal cues. Pitch Patterns offers a unique approach by identifying subtle emotional patterns within speech, providing valuable insights for individuals seeking to improve their emotional intelligence.
By analyzing these patterns, individuals can gauge the effectiveness of their emotional responses and identify areas for growth. Whether it's enhancing emotional regulation, refining empathetic responses, or mastering the art of emotional expression, Pitch Patterns empowers users to become more adept at navigating the complexities of human interaction.
Vision for next decade
Emotions are the lifeblood of human interaction, intricate tapestries woven into the fabric of every conversation. As the architects of our social bonds, they hold the key to unlocking deeper understanding and connection. By deciphering the complex interplay of emotions, we stand at the precipice of a new era of communication, where empathy and resonance become the cornerstones of human interaction.
A future where technology amplifies emotional intelligence is within reach. By investing in the development of sophisticated emotion detection capabilities, we are poised to create a world where interactions are imbued with unprecedented depth of meaning. From the boardroom to the living room, the potential to transform human connection is boundless. Imagine a future where misunderstandings are minimized, trust is fortified, and relationships flourish as a result of our ability to accurately perceive and respond to the emotional landscape.
While 2024 has witnessed a surge in companies like ChatGPT Voice, character.ai, Friend.com, and AI Pin utilizing large language models (LLMs) to craft AI-generated emotional experiences, we believe the true path lies in delving deeper into the intricacies of real human interaction. While the allure of AI-powered emotional experiences, as depicted in films like "Her" and "Blade Runner," is undeniable, it's crucial to recognize the underlying motivation. These portrayals often focus on control, seeking to create systems that exploit human desires for financial gain.
The real opportunity lies in fostering a human-centric approach. Companies and individuals committed to this vision will prioritize understanding and empowering individuals to cultivate greater self-awareness. Instead of passively consuming AI-generated emotional experiences, this approach empowers people to learn about themselves through insightful explanations and a deeper understanding of their own emotional landscapes.
This shift in focus transcends mere financial transactions. By prioritizing human-centered research, we pave the way for a future where technology serves as a bridge, fostering richer, more fulfilling connections with others. Imagine a world where individuals can leverage AI tools to navigate social emotions, fostering healthier relationships and fostering an empowered society.
The 2010s marked the ascendancy of social media and mobile technology, reshaping communication and consumption habits. As we navigate the 2020s and beyond, the integration of AI into media and devices promises an even more immersive and personalized user experience. However, the rapid evolution of technology carries potential pitfalls.
The social media boom of the previous decade is often correlated with a surge in mental health challenges among youth, including decreased attention spans, heightened anxiety, loneliness, and a lack of motivation. These issues raise concerns about the potential negative impacts of the upcoming AI-driven media revolution.
With AI-generated content readily available at our fingertips, critical thinking skills may atrophy as users become increasingly reliant on pre-packaged information. The hyper-realistic and emotionally charged experiences offered by AI could desensitize individuals to real-world interactions, fostering a distorted perception of human relationships. Additionally, the constant pursuit of intensified emotional states through AI-mediated content risks numbing our capacity for genuine emotional connection.
Our antidote lies in rediscovering the ancient wisdom that underpins human flourishing. We turn away from the ephemeral allure of entertainment and pleasure, and instead, focus on the enduring quest for meaning and knowledge. By delving into the core of human needs and learning from the collective wisdom of our ancestors, we seek to illuminate a path towards a more fulfilling existence. Each day is an opportunity to expand our understanding of the world and our place within it, weaving a rich tapestry of experience and purpose.
A future where technology amplifies emotional intelligence is within reach. By investing in the development of sophisticated emotion detection capabilities, we are poised to create a world where interactions are imbued with unprecedented depth of meaning. From the boardroom to the living room, the potential to transform human connection is boundless. Imagine a future where misunderstandings are minimized, trust is fortified, and relationships flourish as a result of our ability to accurately perceive and respond to the emotional landscape.
While 2024 has witnessed a surge in companies like ChatGPT Voice, character.ai, Friend.com, and AI Pin utilizing large language models (LLMs) to craft AI-generated emotional experiences, we believe the true path lies in delving deeper into the intricacies of real human interaction. While the allure of AI-powered emotional experiences, as depicted in films like "Her" and "Blade Runner," is undeniable, it's crucial to recognize the underlying motivation. These portrayals often focus on control, seeking to create systems that exploit human desires for financial gain.
The real opportunity lies in fostering a human-centric approach. Companies and individuals committed to this vision will prioritize understanding and empowering individuals to cultivate greater self-awareness. Instead of passively consuming AI-generated emotional experiences, this approach empowers people to learn about themselves through insightful explanations and a deeper understanding of their own emotional landscapes.
This shift in focus transcends mere financial transactions. By prioritizing human-centered research, we pave the way for a future where technology serves as a bridge, fostering richer, more fulfilling connections with others. Imagine a world where individuals can leverage AI tools to navigate social emotions, fostering healthier relationships and fostering an empowered society.
The 2010s marked the ascendancy of social media and mobile technology, reshaping communication and consumption habits. As we navigate the 2020s and beyond, the integration of AI into media and devices promises an even more immersive and personalized user experience. However, the rapid evolution of technology carries potential pitfalls.
The social media boom of the previous decade is often correlated with a surge in mental health challenges among youth, including decreased attention spans, heightened anxiety, loneliness, and a lack of motivation. These issues raise concerns about the potential negative impacts of the upcoming AI-driven media revolution.
With AI-generated content readily available at our fingertips, critical thinking skills may atrophy as users become increasingly reliant on pre-packaged information. The hyper-realistic and emotionally charged experiences offered by AI could desensitize individuals to real-world interactions, fostering a distorted perception of human relationships. Additionally, the constant pursuit of intensified emotional states through AI-mediated content risks numbing our capacity for genuine emotional connection.
Our antidote lies in rediscovering the ancient wisdom that underpins human flourishing. We turn away from the ephemeral allure of entertainment and pleasure, and instead, focus on the enduring quest for meaning and knowledge. By delving into the core of human needs and learning from the collective wisdom of our ancestors, we seek to illuminate a path towards a more fulfilling existence. Each day is an opportunity to expand our understanding of the world and our place within it, weaving a rich tapestry of experience and purpose.
Emotion characteristics
The basic building blocks of emotions are called affects. These are small negative or positively directed states that we feel, sort of like pleasure or pain. Better analogy would be something that draws as in or something that makes us get away. For instance a person who awkwardly walks into us and smiles while laughingly uttering a heartfelt remark creates curiosity and possible interest, but on opposite side a person who stares at us from distance and utter some angry remarks can make us uncomfortable and shift our attention for ways to manage avoiding these creep who might do something to us.
The next level is the emotion itself, that would be the prolong experience of affects coloured with content, facts and meaning. Thus, a serene spot in nature makes us peacfule and calm as we start to project what we feel, yet the complexity of what triggers it might be hard to explain. Each emotion that you can think of would have some set of charatheristics, but many are universal. Shame for instance will not be the same for everyone, but majority of people within a culture might feel shame of their card is declined when making a payment or when their clothing malfunction.
However, since shame is a more social emotion that might be described as something that you perceive in your own head then it will be much harder to express through tone of voice.
Pitch Patterns identifies emotional patterns in each utterance. Then the agent seeing this patterns can learn if he manage to responde well, or he needs to improve emotion regulation in himself or ability to project emotions better.
The next level is the emotion itself, that would be the prolong experience of affects coloured with content, facts and meaning. Thus, a serene spot in nature makes us peacfule and calm as we start to project what we feel, yet the complexity of what triggers it might be hard to explain. Each emotion that you can think of would have some set of charatheristics, but many are universal. Shame for instance will not be the same for everyone, but majority of people within a culture might feel shame of their card is declined when making a payment or when their clothing malfunction.
However, since shame is a more social emotion that might be described as something that you perceive in your own head then it will be much harder to express through tone of voice.
Pitch Patterns identifies emotional patterns in each utterance. Then the agent seeing this patterns can learn if he manage to responde well, or he needs to improve emotion regulation in himself or ability to project emotions better.
Can emotions be tracked across different languages and cultures?
Yes, our research indicates that speech characteristics associated with emotion recognition exhibit remarkable consistency across different languages. However, it's important to note that our models have primarily been developed and tested on Western languages. To achieve further advancement we recognize the necessity of expanding our dataset to encompass a wider range of languages, including Japanese and Thai
Is emotion recognition in conversation (ERC) reliable?
Yes, based on a multitude of scientific studies, emotions can be objectively measured on positive and negative affect bases (such as happy, sad, angry). Current cutting-edge research focuses on recognizing emotions within social contexts (shame, pride, love, affection, etc.), as these are more context-dependent.
What is difference between basic emotions and social emotions?
Most psychology research focuses on individual psychology, examining the capacity for emotions within a single person. However, a new phenomenon emerges in the context of conversation: what we term "two-person psychology." This arises from the dynamic interplay between two individuals within a relationship, highlighting the social nature of emotions.
Activate Your Sales Team
Achieve growth regardless of your team size. Select the plan that suits your current needs and expand your capabilities when the time is right.
Log In
Not sure? Do you have specific questions? Chat with one of our knights here. You will receive the necessary answers immediately.
Ask the Knight